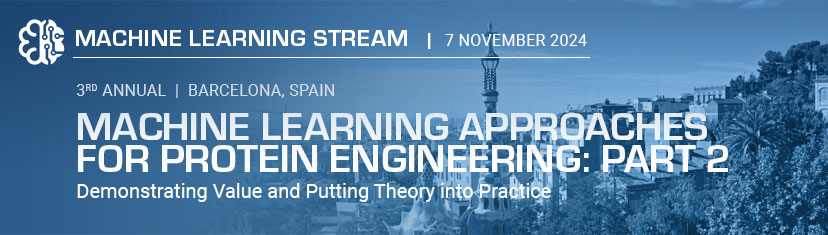
The use of generative AI, deep learning and machine learning tools are poised to make a tremendous impact on the biologics pipeline, but there need to be guidelines put in place to ensure that the results are measurably improved in terms of investment of time, money, and output. Part 2 of the Machine Learning track at PEGS Europe examines and measures the real impact of using these techniques by implementing benchmarks, experimental validation, standards, and controls to guide the process, and case studies comparing traditional approaches with newer, machine learning approaches will shed light on how to adapt and utilize them for biotherapeutic discovery, prediction, developability, simulation and optimization.
Scientific Advisory Board:
M. Frank Erasmus, PhD, Head, Bioinformatics, Specifica, Inc.
Victor Greiff, PhD, Associate Professor, Immunology, University of Oslo
Maria Wendt, PhD, Global Head and Vice President, Digital and Biologics Strategy and Innovation, Sanofi